Guangyi Guo, Ruizhi Chen, Feng Ye, Zuoya Liu, Shihao Xu, Lixiong Huang, Zheng Li, and Long Qian
Published in IEEE Internet of Things Journal (2022), https://doi.org/10.1109/JIOT.2022.3150958
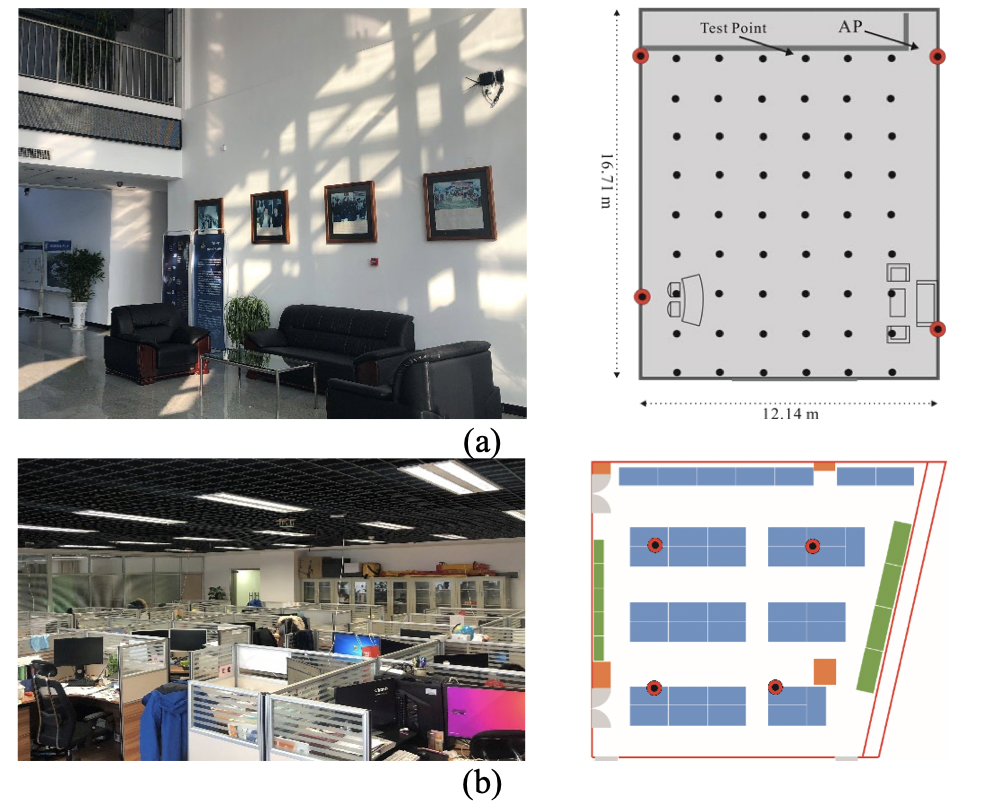
Abstract
As the cornerstone of indoor location-based services (ILBSs), the smartphone-based real-time locating and tracking technologies are now becoming the key for implementing seamless indoor/outdoor location-based applications. Wi-Fi RSS-based positioning system is widely used because of the widespread deployment of Wi-Fi access points in the indoor environment. Correspondingly, the positioning performance of RSS-based method is limited significantly by the complex and time-varying indoor environment. Contrary to the conventional RSS-based techniques, based on the introduction of a two-way ranging approach in the IEEE 802.11-REVmc2 protocol, the Wi-Fi round trip time (RTT) ranging technique provides high-resolution and low-latency ranging observation on smartphones. In this work, a robust integration platform and related positioning algorithms of tightly coupled heterogeneous observables from Wi-Fi and MEMS-IMU are developed for smartphone positioning. The proposed framework optimizes the relative and absolute positioning observables in the integration process and improves the accuracy and stability as compared to the solutions which are based on a single positioning technology. Moreover, the OQECS is established to evaluate the quality of each observation in real-time before feeding the data to the adaptive filter. The experimental results demonstrate that the proposed platform achieves improvement in accuracy and robustness in both real-time tests and simulation tests performed using the polluted data. The average positioning accuracy is 0.572 m, which is 20.22% better than the results obtained from a standard EKF method.